Capital, Green Space, and Race in Salt Lake 2018—A Cross-Sectional Spatial Analysis
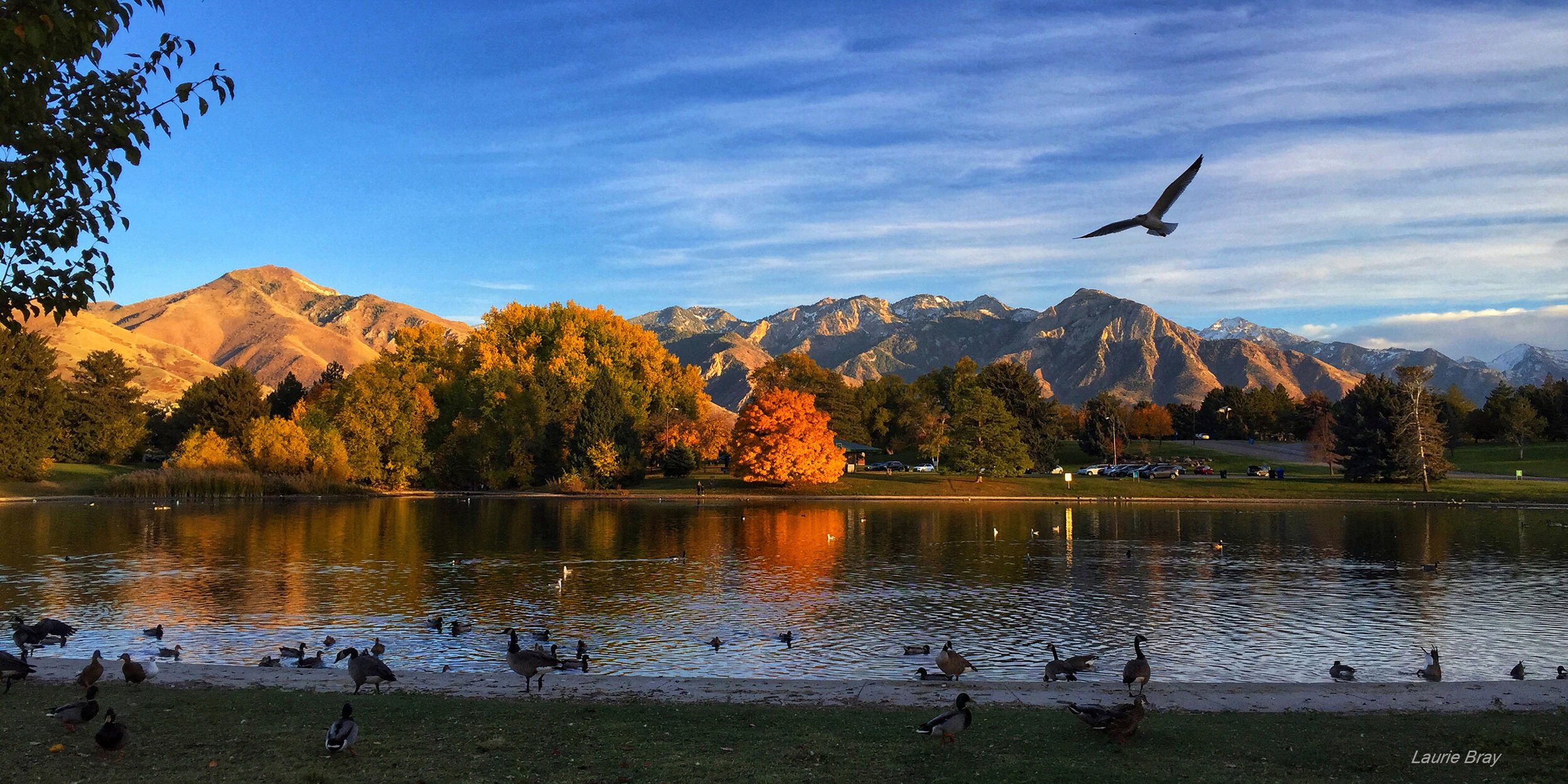
“The term ‘redlining’ is used to describe the practice of limiting access to financial services to people in particular geographic areas and is often associated with the racial composition of a neighborhood.”
BACKGROUND
In the race to provide green amenities in rapidly growing cities, commodified urbanism is set to exacerbate gentrification, and in its wake is a legacy of pushing out the working class who are racially diverse, disproportionately earn less, and lack access to quality education. This investigation provides surface to medium-level spatial visualization and analyzes correlations among green space, measured in normalized difference vegetation index (NDVI), household market values, and the POC and white populations in Salt Lake County, Utah.
While there is ample green space in the county, the majority lie in census tracts with high income, high residential property value, and a high number of white residents predominantly on Salt Lake’s eastside; the opposite is true for the vast majority of POC residents heavily condensed in census tracts with low income, lower residential property value, and little to no green space on Salt Lake’s westside.
As cities urbanize, the demands for mobile transport, sustainability, and green space rise with it, and while some calls spur discourse and enter policy, many developers and individuals take it upon themselves to purchase land and alter property in whichever neighborhood they’re invested in or live in, respectively. Environmental degradation already has massive repercussions, and more projected, against minorities (e.g. queer communities, POC, working class), and in the process answering sustainability at the white and/or wealthy’s behest (i.e. NIMBY and YIMBY), it frequently results in environmental and social inequity against the ones already at the margins of legislation and city planning.
Study Area
This investigation provides analyses on Salt Lake’s census tracts that show green spaces, finding whether or not there is a correlation among them with variables like household market value, median household income, the POC population, and the white population.
If there happens to be a positive relationship between capital and green space, then is there a correlation with racial disparities?
REDLINING AND NO LINING—RACISM AND PLANNING DISCRIMINATION
The provided map shows the redlined and no lined areas (created in the 1940s) in roughly the Salt Lake City region, where they are overlaid with the peripheral neighborhoods known today, which were informed by Reddit discussions and “most dangerous neighborhoods” rankings in Salt Lake.
Anecdotal Note: As a local who’s lived in the westside and the opportunity to be able to live in the eastside as well as frequently commuting to the northwestern regions in the map (e.g. Rose Park, Glendale, Poplar Grove), I’d personally argue that Liberty Wells isn’t a peripheral neighborhood compared—I only imagine it’s viewed that way because many of the unsheltered were pushed out due to the Rio Grande project and other anti-homeless initiatives, and many created tented areas in Liberty Wells and southern Central City (e.g. Liberty Park).
Redlining was a discriminatory process borne from the New Deal era during the 1930s to curb foreclosures and stabilize the economy in the wake of the Great Depression, and these attempts resulted in federal aid programs offering government-insured mortgages; these financial solutions compelled predominantly white real estate to exclude non-white races—this racial steering of different regions in cities would later create quarters densely packed with BIPOC and working class without upward social mobility, effectively oppressing and suppressing their presence, and physically restricting them to ethnic and cultural enclaves on the outskirts, where they’d have to struggle to build on the margins of policy. No lining was another racially driven action that attributed some places as industrial, or omitted entirely, when in fact there were people living within the parts.
The redlined are people who disproportionately suffer from degrading environmental hazards, inhumane work conditions, meager to no educational accessibility, lower income, and other restrictions that would affect generations to come—and the first targets, as is the tendency, were Black, Hispanic, and Latina/o populations, and then other POC. Today, it’s almost guaranteed that these unwanted communities are still primarily condensed in these redlined zones where the Home Owners’ Land Corporation (HOLC) deemed them “hazardous” and warned away any prospective investment within those areas. Areas like Salt Lake’s Chicana/o communities, other Hispanic ethnicities, Black, and AAPI (Asian American and Pacific Islander) populations in the county’s westside; the segregation of Black communities to Dorchester, Roxbury, and Mattapan in the Boston area; Dorchester’s Little Saigon with thousands of Vietnamese refugee migrants from the Vietnam War; and Oakland, California’s dense Black, Asian, and Hispanic / Latina/o demographic.
REDLINING’S UNQUANTIFIABLE DAMAGE
Those in redlined areas and outside of them often refer and speak of each other with contempt or derision, whether it’s charged statements about the margins being inherently criminal, violent, and engaged in drug-use when the white-collared fostered the long-standing inequality in the first place, with which many in the periphery show profound and understandable indignance toward.
Of course, redlining’s legacy doesn’t stop in Salt Lake or Oakland or Boston or wherever else; while the practice aimed to disinvest the negatively graded districts, many previously redlined parts are seeing gentrification encroaching upon their municipal boundaries. Neighborhoods that were economically depressed were revitalized by shunned populations, and it’s only then that modern developers and real estate recognize value in these localities, where they see monetary potential rather than cultures flourishing despite the hardships forced upon them by these very same gentrifiers.
The land—and only the land—in which the deprived and dispossessed reside, is valuable in the face of urbanization.
Make no mistake, the undesirables remain undesirable.
METHODOLOGY
Existing, open-source data was collected and uploaded into ArcGIS to map out census tracts, and graphs were created using Exploratory.
Census Tract Boundaries (2018 ACS 5-Year Estimate): Census tracts in Salt Lake, polygon vectors symbolized with black outlines.
Parks (Utah AGRC): Parks in Salt Lake, polygon vectors symbolized by blue, green, and pink shades depending on visibility needs.
Peripheral Neighborhoods (Tessa Vu): Neighborhoods in the margins of Salt Lake, polygon vectors symbolized with black outlines and dotted shading. Data by a local to Liberty Wells who has also lived in Kearns, West Valley, Cottonwood Heights, Millcreek, and Sugarhouse, and commuted often to Glendale and Rose Park. Created with both anecdotal knowledge and from a list of Salt Lake’s most “dangerous” neighborhoods.
Main Roads (Tessa Vu): Busiest streets and highways.
Normalized Difference Vegetation Index / NDVI (class data from Dr. Andy Hong): Mean NDVI values in Salt Lake, symbolized by graduated brown-green colors with numbers ranging from below 0.228167 to 0.555407. They are sorted lowest to highest (lightest to darkest) and categorized: sparsest (≤ 0.228167), sparse (≤ to 0.309977), moderate (≤ 0.391787), healthy (≤ 0.473597), and healthiest (≤ 0.555407). The five classes were split using natural breaks and labeled according to common practices.
Population Data by Race (2018 ACS 5-Year Estimate): All symbolized by graduated orange-red colors, sorted lowest to highest (lightest to darkest), normalized dividing by the total population (i.e. what percent of a specific race makes up a census tract), and split into five classes using natural breaks: Sparse, Low, Moderate, High, and Dense.
White population after normalization range from below 0.573075 to 0.981051: ≤ 0.573075, ≤ 0.73523, ≤ 0.832761, ≤ 0.901275, and ≤ 0.981051. Note that even the break-off for a “sparse” white population is 57.3075% of a census tract’s population.
Black population after normalization range from below 0.009218 to 0.156725: ≤ 0.009218, ≤ 0.025947, ≤ 0.049573, ≤ 0.100073, and ≤ 0.156725. Note that the densest Black communities only make up 15.6725% of a census tract’s population.
Hispanic / Latina/o population after normalization range from below 0.074779 to 0.607001: ≤ 0.074779, ≤ 0.149275, ≤ 0.261029, ≤ 0.408757, and ≤ 0.607001.
Native American population after normalization range from below 0.004176 to 0.085517: ≤ 0.004176, ≤ 0.012543, ≤ 0.026367, ≤ 0.051414, and ≤ 0.085517. Note that the densest Native American communities only make up 8.5517% of a census tract’s population and the lowest being near zero at 0.4176% of a census tract’s population.
Asian population after normalization range from below 0.021163 to 0.219237: ≤ 0.021163, ≤ 0.040438, ≤ 0.064222, ≤ 0.114794, and ≤ 0.219237.
Pacific Islander population after normalization range from below 0.006183 to 0.135329: ≤ 0.006183, ≤ 0.017764, ≤ 0.034377, ≤ 0.061473, and ≤ 0.135329.
Other race population after normalization range from below 0.035429 to 0.460949: ≤ 0.035429, ≤ 0.089147, ≤ 0.15816, ≤ 0.274232, and ≤ 0.460949.
Two or more races population after normalization range from below 0.016751 to 0.118982: ≤ 0.016751, ≤ 0.028897, ≤ 0.043685, ≤ 0.067941, and ≤ 0.118982.
Capital-Related Data (2018 ACS 5-Year Estimate): All symbolized by graduated colors, sorted lowest to highest (lightest to darkest) and split into five classes using natural breaks: Low, Moderately Low, Moderate, Moderately High, and High.
Median household income, symbolized by graduated yellow-blue shades with numbers ranging from below $52,547 to $203,194: ≤ $52,547; ≤ $71,597; ≤ $92,799; ≤ $120,230; and ≤ $203,194.
Median market values of houses, symbolized by graduated purple shades with numbers ranging from below $204,500 to $822,500: ≤ $204,500; ≤ $283,200; ≤ $372,600; ≤ $496,400; and ≤ $822,500.
Median gross rents, symbolized by graduated pink shades with numbers ranging from below $977 to $2,500: ≤ $977, ≤ $1194, ≤ $1427, ≤ $1733, and ≤ $2500.
RESULTS
The green space map above shows that the majority of the denser areas are located in Salt Lake’s easternmost side as it joins the Rocky Mountains. There are also bare spaces in census tracts along the I-15 highway that cuts through South Salt Lake and rides along the boundaries that join Ballpark (also known as People’s Freeway), Central City, Glendale, and Poplar Grove. There is also a visual correlation between the amount of parks and the NDVI levels of each census tract. To note, 2018 saw many major construction and reconstruction of interstates as well as state routes such as Bangerter, the 201, I-215, I-15, and I-80, among more.
The income map above shows that many of the census tracts that are higher-income tend to match with the denser census tracts of the green space map. There is also a stronger correlation between parks and household income as opposed to the previous map. Income may also be lower in the census tracts around highways, which have less residential development traveling toward the city center and west, though caution should be exercised as this project doesn’t discern whether or not these spaces are for public recreational use, which is a different study.
The house value map above shows a visual correlation between property values and green space. The prices are unsurprisingly higher on the east and northeast sides since many low-density houses and older buildings were constructed on the cusp of the mountains. This is contrasted to the westside and southwest side, which are both victims of urban sprawl and are rapidly suburbanizing into cookie-cutter neighborhoods and newly-erected apartment complexes. The gross rent map is more representative of this expansion as jurisdictions are leaning towards high-density lodging in partnership with developers, which has interesting future implications that need to be investigated seeing as Utah is one of the least renter-friendly states in the U.S.
In addition, there is a patch of census tracts in the southwest side that are darker in the median income and gross rent maps that can be attributed to the fact that it’s a predominantly wealthier pocket (they have a man-made island with suburbs and a high concentration of LDS churches). While there are low-density houses in these Daybreak and Country Crossing areas, they are dominated by renters.
Categories: 57.3075%, 73.523%, 83.2761%, 90.1275%, and 98.1051%.
White individuals, despite making up more than 86% of the entire county’s population at the time, primarily concentrate in census tracts that are either higher in property value and gross rent, and by extension higher green spaces in the south and west sides. They are very much not as dense in the peripheral neighborhoods.
Categories: 0.9218%, 2.5947%, 4.9573%, 10.0073%, and 15.6725%.
This population map shows that Black communities are concentrated in census tracts that are either lower in property value and gross rent and possess lower household income, by extension being more distanced from green spaces. The communities are clustered around the city center, Ballpark, and Glendale, and sporadically on the westside—this is expected as Black communities create ethnic enclaves which are often isolated and less spatially integrated to other races, a trend also shared by the Vietnamese in West Valley and Kearns whereas Chinese, Japanese, and Koreans tend to be more spatially integrated to white populations compared.
Categories: 7.4779%, 14.9275%, 26.1029%, 40.8757%, and 60.7001%.
Anecdotally, if a local were to describe the demographic of the westside, they would undoubtedly mention it has a very dense Hispanic or Latina/o population—the largest ethnicity being Mexicans during the Mexican Revolution and agricultural expansion where many sought to find work within the industry. This influx of immigrants, both Hispanic and Chinese, eventually led to redlining the county, which is why many POC, especially Hispanic and Black communities, are on the receiving end of disproportionately lower income, education, and skilled work.
As a result, a great portion of the westside has an enduring Chicana/o population that developed many neighborhoods’ barrio urbanism as the people settled, urbanized, and suburbanized.
Categories: 0.4176%, 1.2543%, 2.6367%, 5.1414%, and 8.5517%.
The Native Americans share a somewhat similar spatial distribution to the Black community, the greatest concentration in Ballpark, which is expected as the Urban Indian Center of Salt Lake is located there. Another high concentration is just east outside of South Salt Lake, presumably because the community could be gravitated toward one of the only Native American restaurants in the entire county, Navajo Hogan—this could also explain the dense patch in West Valley City’s southeast corner as it contains the Native American Trading Post that sells goods from the indigenous peoples of Utah.
Categories: 2.1163%, 4.0438%, 6.4222%, 11.4794%, and 21.9237%.
The Asian demographic is spatially varied, which is expected due to the Asian-American diaspora possessing the largest wealth gap of all races in the United States that’s furthered class and ethnic tensions within the overall community. The top three ethnicities in order are Chinese (8949), Indian (7674), and Vietnamese (7283) in 2018, historically due to several push and pull factors like wars and other violent conflict in their countries, the Gold Rush, filling unskilled labor, etc. This distribution resulted in many cultural enclaves within Salt Lake surrounding Indian restaurants and grocers, the Chinatown market, businesses like salons, and ethnic churches outside the LDS sphere.
Disproportionately lower income, education, and skilled work also plague the Southeast Asian demographic, which is a large gap when observing within Asian-American ethnicities, but a lesser degree compared to the Hispanic and Black communities in Salt Lake.
Categories: 0.6183%, 1.7764%, 3.4377%, 6.1473%, and 13.5329%.
The islander population is also spatially varied, though not as intensely as the Asian demographic. Historically, the LDS church has strong links with Polynesia and Hawai’i, many missionary efforts were exerted in the islands and resulted in cultural pockets in Salt Lake, either integrated within their own enclaves or around the LDS religion. However, the early immigrants were told to create communities in the westside, hence, the densities in that area, but after some towns fell and were abandoned, many subsequently returned to their islands or spread elsewhere in Salt Lake.
Categories: 3.5429%, 8.9147%, 15.816%, 27.4232%, and 46.0949%.
This category in the census is primarily made up of Brazilians, Guyanese, Cabo Verdeans, Belizeans, and Mauritanians. Note that while the article notes that the ACS posted these distinctions for the first time in 2023 based off of the 2020 census, this project is assuming races that filled out “Some Other Race” for that year likely did it previous years.
It looks as the spatial pattern is very similar to the Hispanic / Latina/o distribution. This is an assumption, but it could be due to the fact that Brazilians make up a vast majority of the “Some Other Race” category in the census and could have integrated with the Hispanic community due to linguistic similarities and shared histories in unskilled labor opportunities, along with the other majorities in the category—another factor is a portion of these demographics are converts into the LDS faith.
Categories: 1.6751%, 2.8897%, 4.3685%, 6.7941%, and 11.8982%.
With diversity often comes the integration of many races, and despite Salt Lake County being saturated with a white population over 86% at the time, many POC communities as well as white communities inevitably integrated. The spatial variety is expected for the “Two or More Races” category, and it’s unsurprisingly more clustered in areas that have a higher density of POC and greater diversity, which explains how the census tracts with two or more races almost bleed out into areas with a high percentage of white individuals.
Some Terminology in Brief
The dotted line and shaded area around it is what is called linear regression, where a mathematical equation is used to predict two variables.
It calculates several values, the four shown being Correlation, R-Squared, Coefficient, and P-Value, but the Coefficient need not be covered.
Correlation values are between -1 and 1. -1 indicates complete negative correlation meaning that when one variable goes up the other goes down the same degree. 1 indicates complete positive correlation meaning that when one variable goes up the other goes up the same degree. 0 is absolutely no correlation. When realistically applied, there will rarely be a perfect negative, positive, and rarely no correlation either (read: correlation values will usually range from -0.99 to -0.01 and 0.01 to 0.99, but even those maximum and minimum values aren’t seen often).
R-Squared, also known as R² or the coefficient of determination, is a statistical measure in a regression model, in this case a linear regression model, that determines whether the dependent variable’s (average household value) pattern can be explained by the independent variable (green space) influencing it—often referred to as the goodness of fit.
P-Value ranges from 0 to 1, and is the probability that someone would obtain the effect observed in the sample, if the null hypothesis is true for the populations the number would be closer to 1—p-values are calculated based on the sample data and under the assumption that the null hypothesis is true. Lower p-values indicate greater evidence against the null hypothesis. The null hypothesis is that there is no causative relationship between the variables (read: assuming no correlation).
NDVI and Income: Correlation = 0.66627958931; R-Squared = 0.443928; P-Value = 2.17422e-26.
NDVI and House Value: Correlation = 0.56007574121; R-Squared = 0.313685; P-Value = 1.69877e-17.
NDVI and Gross Rent: Correlation = 0.46361596072; R-Squared = 0.214940; P-Value = 1.26973e-11.
Observing the variables in the linear regression, the strength of all three values are ranked from highest to lowest NDVI’s relationship with income, house value, then gross rent. However, correlation is not considered strong unless the value is greater than 0.70 or 0.75, of which none of the variables breach—this lack of solid correlation is expected in an urbanizing area that isn’t prioritizing creating tree canopies, though not to say it isn’t being planned; the focus lies on high-density development, which could be linked to the NDVI and gross rent relationship as demand for housing precedes constructing green spaces, though that is an anecdotal observation that should be investigated separately.
On the side of ownership and low-density housing, it is expected that it has a stronger positive relationship due to much of eastside property being proximal to green space (e.g. Rocky Mountains, outdoor activities) as well as single-family homes generally having larger land area and yards. NDVI and income has the strongest positive relationship out of the three dependent variables, while green space may affect property and rent, it could suggest that those with higher income tend to prefer nearness to green spaces and could possibly be affected by more expensive outdoor sports and activities such as golfing.
Regarding the R-Squared values, the fact that all numbers are under 0.50 indicates that the linear regression provided a line of best fit that is not suitable to use as a predictor, which is expected due to the intrinsically socially varied nature that revolves around capital and green space. The P-Values being near 0 indicates evidence against the null hypothesis (i.e. essentially no correlation, so in this case it would be that “NDVI, or green space, has no affect on capital”). So, it is highly unlikely these values are due to chance.
The following graphs are symbolized by yellow-green-blue graduated colors (lowest to highest being lightest to darkest color-wise and smallest to biggest size-wise) with the NDVI independent variable on the x-axis and the capital-related dependent variables on the y-axis (income, house value, gross rent), and are proportioned according to the different races, providing a 2.5D visualization.
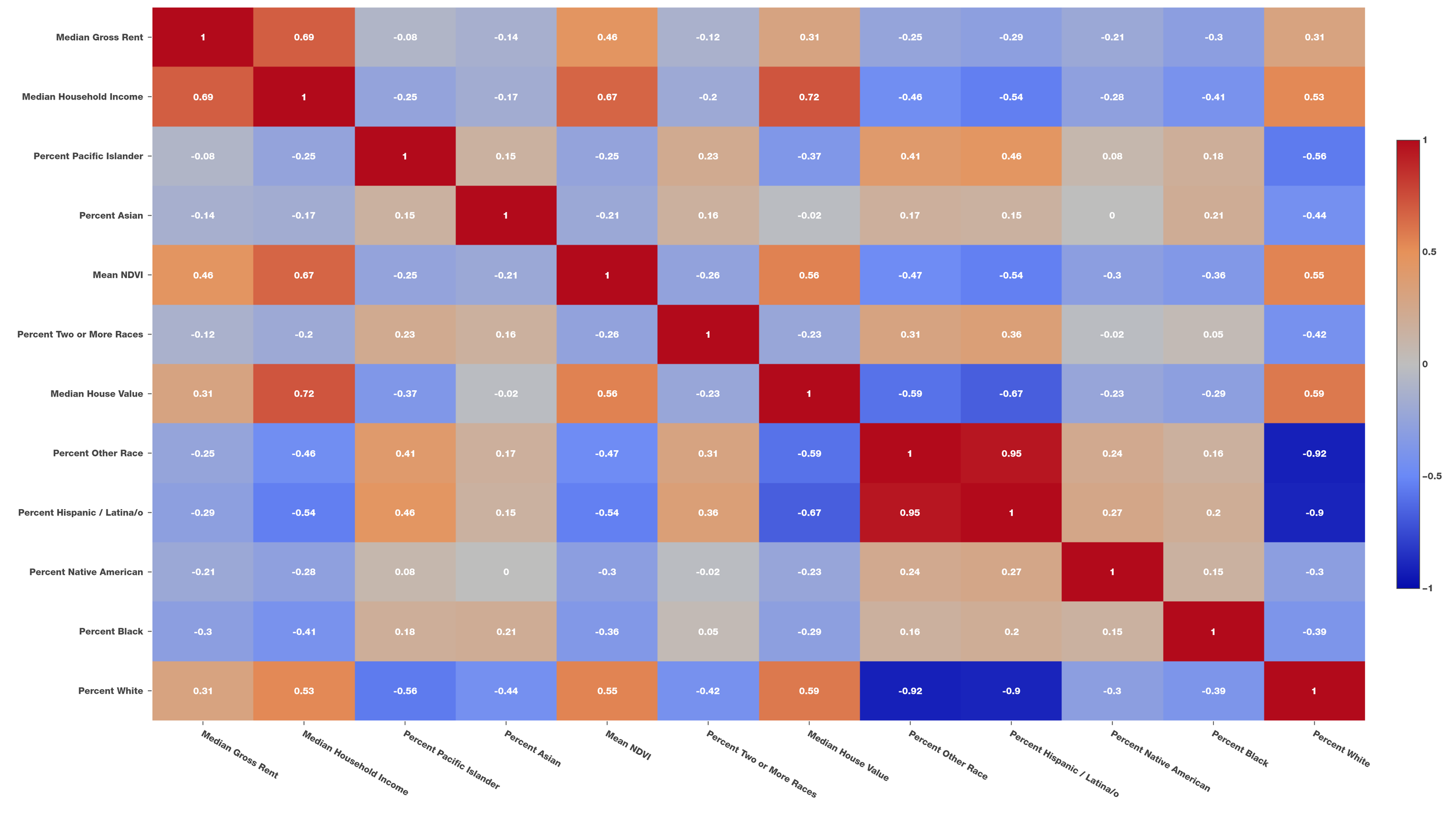
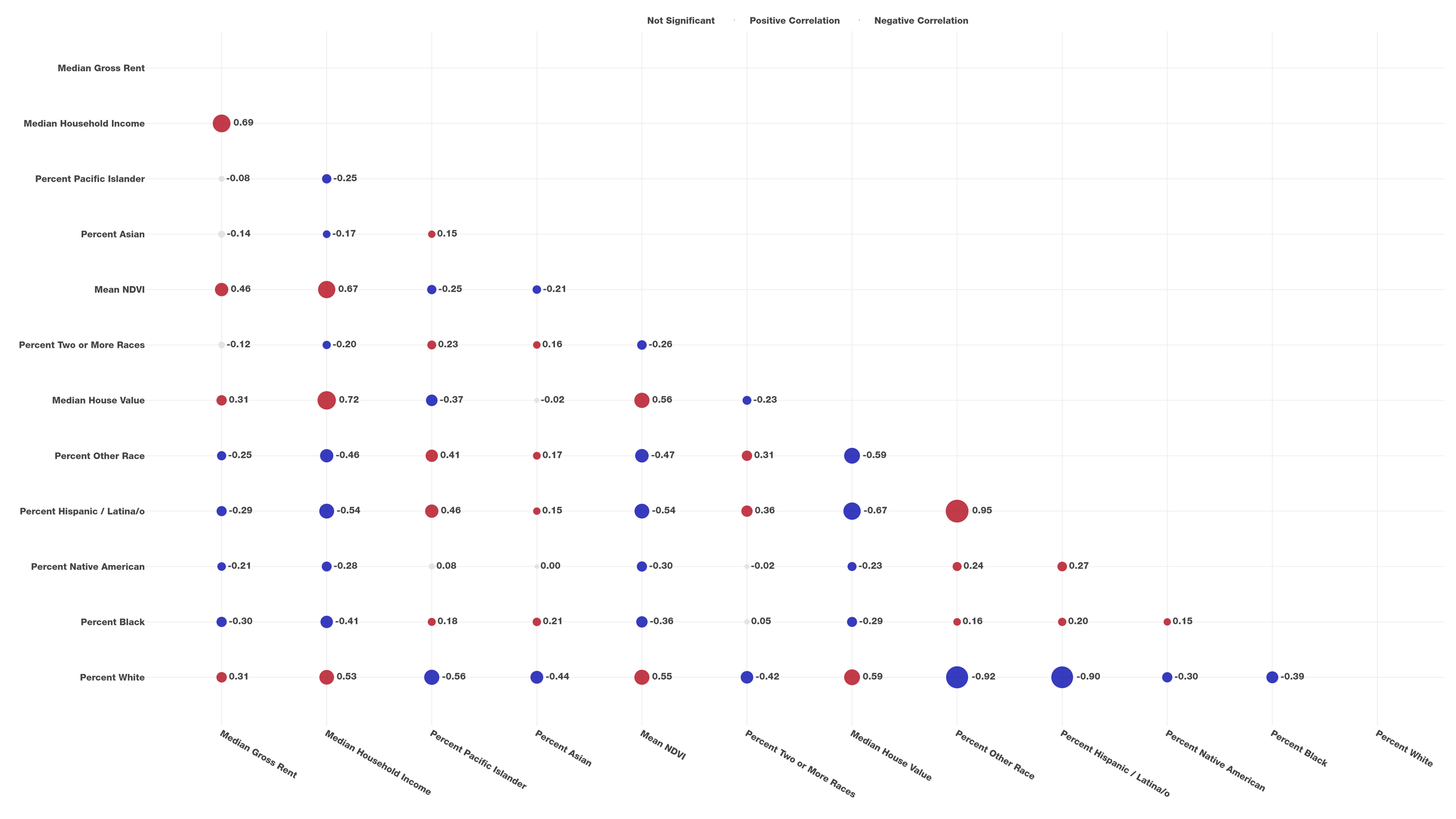
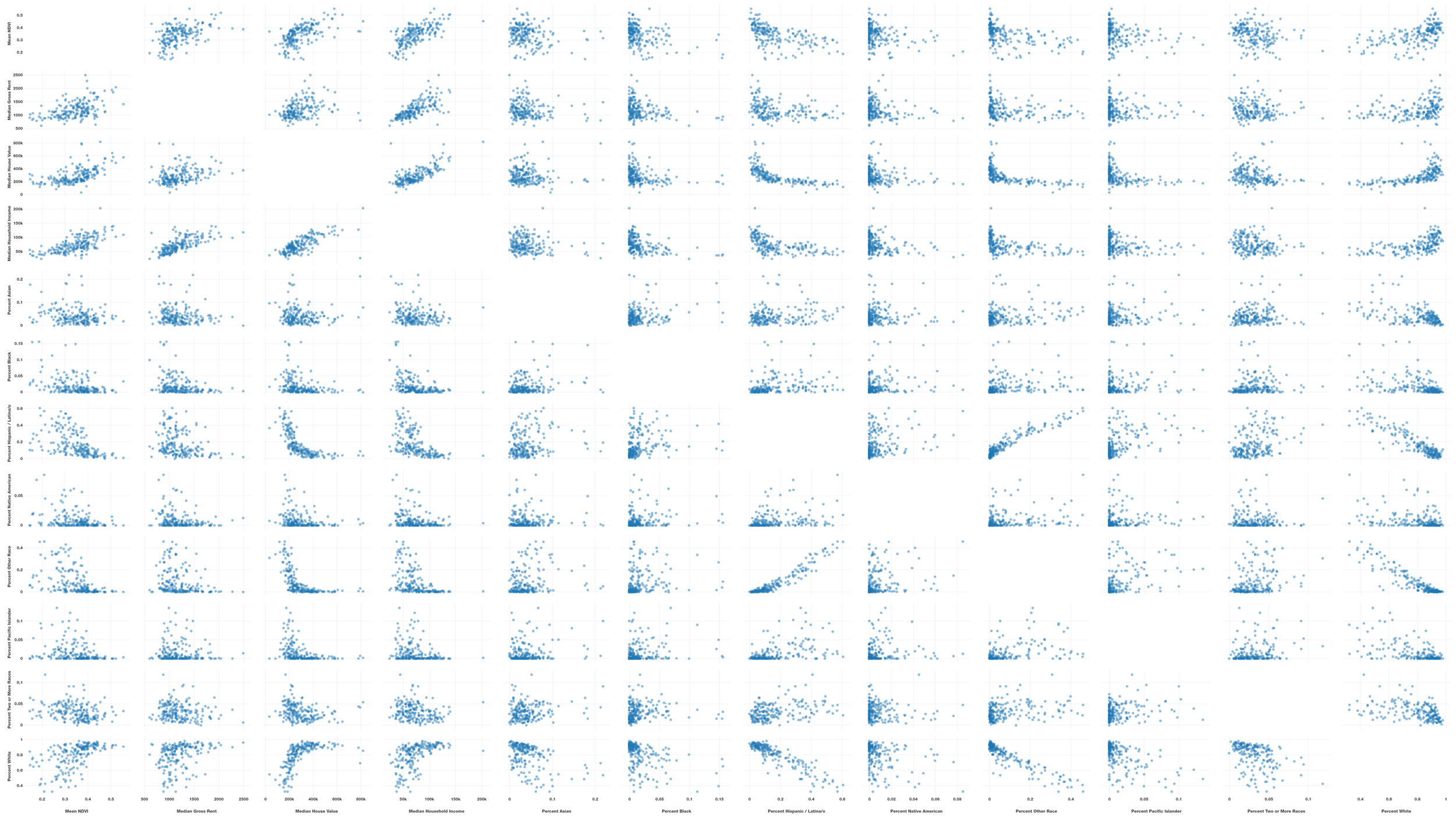
The above graphs measure the correlation among all variables that were observed in this investigation.
The white population is the only demographic that has all positive relationships with NDVI and capital, and it suggests they are the least integrated (negative values) with, in order, Other Races, Hispanics, Pacific Islanders, Asians, Two or More Races, Black, and Native Americans. All POC are either lightly or somewhat integrated (the highest between Hispanics and Other Races), although an index of dissimilarity would be needed to truly analyze spatial integration for all races—even if they may live within the same census tract, they may have created enclaves within the areas and seldomly interact.
Something else to note is that the Native American population resulted in many correlations considered “Not Significant”, likely due to the fact that they make up the least numbers in Salt Lake’s population, the race after being Black, so the sample numbers were not high enough to measure correlation.
PROBLEMS
While this study simply examines cross-sectional data at a single point in time, the analyses derived from the figures should not be overlooked, and further investigations should be made at the finer level among ethnicities alongside temporal data. Another concern is that some of the data is not recorded in some census tracts and may have shifted the figures (e.g. NDVI data). In a future study, it could be worthwhile to also include capital values for commercial, business, and religious constructs in Salt Lake as well as zoning and redlining.
There are also variables unaccounted for that could potentially affect some datasets such as air pollution, construction zones, park usage, and especially unsheltered communities. Something to note is that green spaces, if not used for recreational means, may be within opportunity zones, and are therefore “undeveloped” (read: to be developed and gentrified, or potentially made into a public outdoor space that may or may not be a LULU).
FINAL THOUGHTS
After comparing the maps it is easy to see there are some strong positive correlations among several variables, specifically NDVI and capital data, as well as spatial correlations with POC and said data. While there is not sufficient enough data to draw causation, especially due to the preliminary nature of this analysis, there is enough data to imply it. This should prompt further studies in gentrification, displacement, and equity within nascent metropolises like Salt Lake.
With that, a solution that could alleviate dispossessing marginalized groups and the worsening inequity is—and this is stated ad nauseam time and again—
“Working with legislation to enact equitable green policies.”
Or the like.
However, peaceful protesting has been ineffective.
The statement isn’t meant to deter nor undercut historical figures behind that movement to fight against injustice.
Harmonious methods against a larger, systemic apparatus that’s sowed violent and invisible discord often meanders behind the front lines of, for example, grassroots organizations with passionate, marginalized individuals who demand and disrupt because they won’t be heard otherwise.
It seems peaceful protesting is the ideal example to fall back on when disruptive methods interfere with the everyday, when capital is disturbed and then neo-liberal outcries undermine the efficacy of said methods.
Those on the outskirts of policy only grow with bloating cities, worsening the existing socio-economic inequalities. Those attempting to implement laws and regulations concerned with urban sustainability often become gentrifiers because these are neighborhoods physically removed from their everyday, and they know it—there may be a wide variety of stakeholders, but the ones with the final say are developers and government who revolve their focus around capital. The only way to de-gentrify and create a truly just city is to decolonize the infrastructure, whether that is supporting grassroots endeavors, or replacing the current commodifying mindset with those who want to achieve equity in spaces dominated by the former.